Hello everyone and welcome to this week's blog post. Today, I'm going to be exploring in detail how artificial intelligence (AI) is revolutionising medicine and patient care. For the non-scientists reading this week's post - don't worry - I will make sure any of the computer science terms I use are simple and easy to understand!
Before I continue with the rest of the article, I'm going to quickly define some of the terms that I will be using and describe in simple terms how AI works.
Computer algorithm: a series of step-by-step instructions which are followed by a computer to carry out a task. A computer program usually outputs the result of the algorithm so that it can be used by a human.
Artificial intelligence: any computer program that performs algorithms which would normally need human intelligence to carry out. An example of this would be speech recognition or visual perception.
Machine learning: A subset of artificial intelligence, which uses a different kind of algorithm. Instead of the step-by-step instructions being written by humans, the instructions are actually figured out by the computer. In this type of artificial intelligence, the computer is trained to recognise patterns which it then uses to complete the task.
Deep learning: A subset of machine learning which uses deep neural networks (I'll explain what these are later) to train an algorithm.
One of the biggest areas of diagnostic medicine that AI can help in is the diagnosis of cancer, as it can drastically reduce the time taken for a scan to be reviewed, as well as reduce the risk that the cancer is missed by the clinician.
The vast majority of cancer-recognising programs use supervised learning to train the algorithm. Supervised learning strives to learn the relationship between given inputs (such as features recognised on a scan) and a single output (such as whether a patient has cancer). The way it works is that humans begin by labelling the input data - this is called training data. In the case of cancer recognition, this takes the form of a doctor reviewing a set number of scans and annotating them with features that they look for when deciding if there is cancer (such as a large mass which displaces the surrounding tissue. They also record whether cancer is present or not. The algorithm then receives this data and passes it through an artificial neural network to develop an algorithm.
An artificial neural network (ANN) works in similar ways to the human brain. Just like in the brain where everyday connections are made, strengthened or weakened between neurons, the same process occurs in a neural network. An ANN can contain up to millions of artificial neurons, called units or nodes, arranged in a series of layers (see below).
To begin with, generally, all nodes are interconnected with every node on the layers either side of it. Then, the training data is input one by one into the input nodes (each feature of the image is given a different node). The data then passes through the layers until an output is produced. After the output is compared to the expected output (whether the patient has cancer or not), the strength of each connection between nodes is altered. More training data is input, and the nodes are continuously adjusted until any more adjustment of the nodes results in the output becoming less accurate. At this point, the algorithm needed has been trained. As such, an image can then be input into the program without labelling, and the program would be able to accurately deduce whether the scan showed signs of cancer.

The implications of this for diagnosing cancer are immense and far-reaching. Not only does this mean that places which don't have the funds to employ a radiologist still can work out whether a scan shows signs of cancer. Furthermore, a cancer-recognising program could also improve patient experience and the percentage of cancer cases that are spotted. Not only can scans be reviewed more quickly, but studies suggest that they may be better than doctors at spotting cancer. Besides, unlike doctors, computer programs never tire, meaning that a high level of accuracy is always maintained.
A study published in January 2020 showed how powerful these scans can in diagnosing cancer. In the study, a team including researchers from Google Health and Imperial College London were able to train a computer model that could recognise breast cancer in scans better than six radiologists. Indeed, using the software saw a reduction of 2.7% of cases where cancer is missed (a false negative).
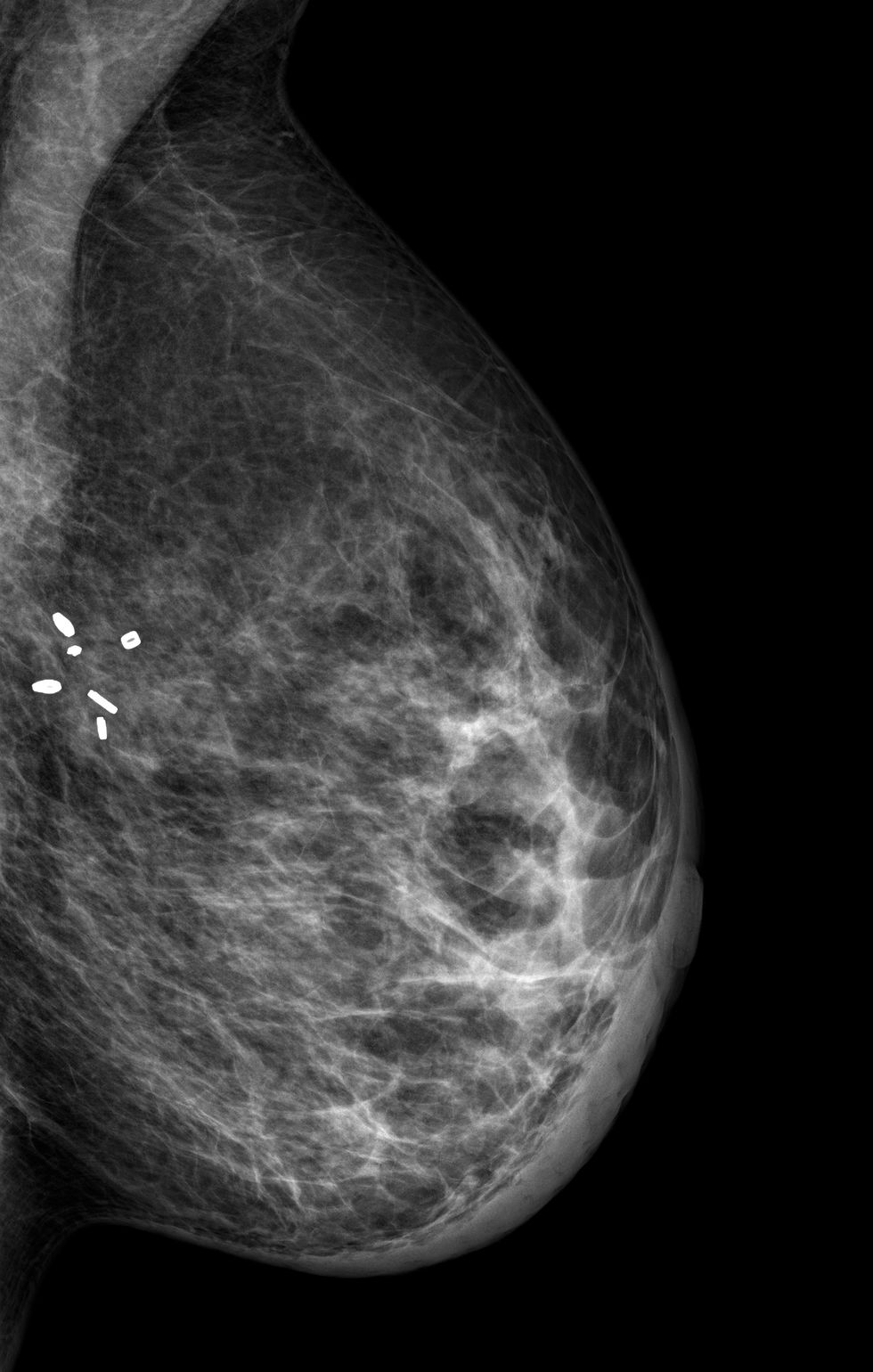
Nevertheless, one must be wary of the fact that everyone's anatomy is different - as such one can't be sure that a tumour is present, even if the program states that there is. Furthermore, particularly with regards to cancer, the result is not always definitive. A scan can only tell you if a tumour is present, not whether the tumour is benign or cancerous. As such, a doctor is still needed to perform other tests (such as biopsies) to confirm a diagnosis. Also, developing machine learning algorithms can be expensive, especially when considering how much time is needed for experts to annotate training data. Nonetheless, you could say that this cost is more than offset by the fact that cancer is spotted earlier, meaning that less intensive care is needed. The life-saving potential of these algorithms also more than offsets these economic considerations.
The other main area of diagnostic medicine which uses AI are symptom checkers. These are AI-powered programs located either online or on apps which ask patients questions about their symptoms and use their answers to guess the most likely illness the patient has. There are many platforms which do this - for example, the new NHS app includes this feature. While these technologies do some promise and should improve in the future, there are several concerns associated with them. Firstly, while they usually get better results than simply typing in symptoms into Google, many often miss life-threatening illnesses. For example, according to Which?, the Ada app completely missed the diagnosis of meningitis. On the other hand, the NHS app errs on the side of caution, suggesting that insomnia patients seek advice as soon as possible. Secondly, as patients have to describe their own symptoms, some symptoms may be incorrectly described, and some might be missed altogether. This could mean that patients could either get referred to emergency services when it's not necessary or delay seeking treatment until its too late.
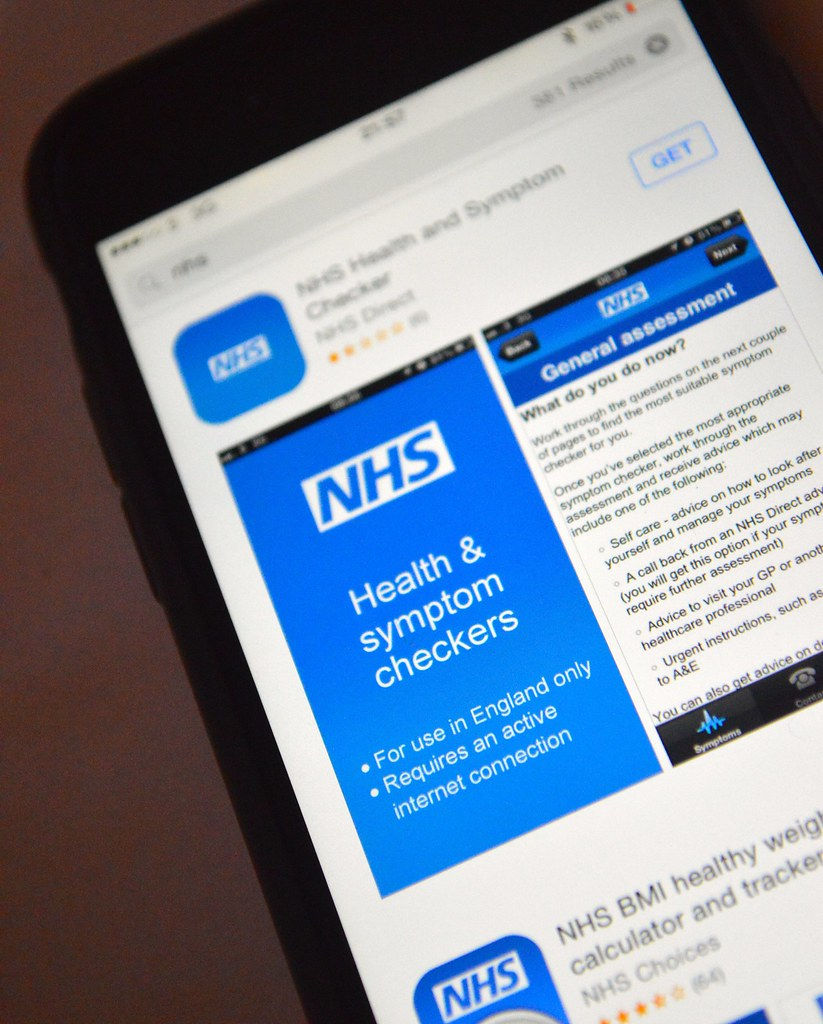
As such, while in the future these AI-powered symptom checkers may prove to be more effective, I would contend that for the moment it is too risky to rely on them. While some time could be saved, as patients with minor illnesses might not visit A&E, this doesn't outweigh the potential risks of the program missing the illness altogether. Furthermore, the economic argument in that it saves time also doesn't hold as more patients could be referred to a hospital without having any serious conditions, thus wasting valuable hospital resources.
In conclusion, the future of medical AI in the UK looks very promising, especially with the UK government embracing this new technology. In August 2019, the UK government announced that it would spend £250 million in setting up an NHS AI lab. This lab aims to develop algorithms to diagnose disease, as well as algorithms to predict hospital bed demand to reduce strain on healthcare services. Nevertheless, while these developments in medicine look promising, we must remember that just because a program has the words "artificial intelligence" in it, it doesn't mean that it works perfectly. This is particularly notable with the rise of AI-symptom checkers on the internet, which don't have to be regulated and could give misleading information.
Sources:
Comments